Neuromorphic Technologies
Currently, artificial intelligence technologies are showing impressive results capable of changing the world order. Existing computing systems have limitations in performance, energy efficiency, and scalability of classical computing systems.
The key to overcoming these barriers is the transition from the classical von Neumann architecture to bio-inspired, neuromorphic architectures that allow organizing vector-matrix multiplication in memory and ensuring massive parallelism of computations.
One of the most promising approaches to the hardware implementation of artificial neural networks and neuromorphic systems is the use of electronic components based on memristive devices and memory based on them (ReRAM). Research results confirm that systems based on memristive devices have advantages over solutions based on traditional electronics (FLASH, CPU, GPU, TPU, FPGA).
Acceleration of Neural Networks
- Massive parallelism
- Computations in memory
- Training on data streams
Neuromorphic Properties
- Transmission of signalstied totime
- Potential biocompatibility
- Spike signal transmission
Energy Efficiency
- 100 times more energy efficient than Flash memory cells
- Low currents, lowvoltages
- Non-volatile data storage
Durability
- Data retention time over 10 years
- Cell endurance 1 million times more write cycles than Flash memory cells
Performance
- Data placement density 10 times higher than Flash cells
- Read speed 100 times faster
- Write speed 1 million times faster
Scalability
- Target scale 100nm
- Proven scalability of technology 28nm
- Possibility of further scaling <22nm
Extreme Operating Conditions
- High temperatures
- Resistance to EMI
- Resistance to radiation exposure
Cost
- Cost comparable to manufacturing Flash cells
- Standard materials
- Unified production process
About the Product
Our mission is to develop Russia as a leader in neurotechnologies and artificial intelligence by creating a convenient platform for developing innovative solutions. To offer the best conditions to partners and employees, striving to achieve maximum success in the development of the company and the industry.
We are creating an advanced ecosystem for working with neuromorphic systems, including EDA (Electronic design automation) and hardware tool. Our goal is to make neuromorphic technologies accessible to school students, university students, engineer enthusiasts, and professional developers.
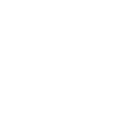
EDA
Our Electronic design automation (EDA) system allows you to model, design, and test neuromorphic devices within a unified software environment. It simplifies development by minimizing errors and accelerating the transition from concept to finished solution. The EDA is created in accordance with the best global practices and is compatible with modern software packages and industrial standards.
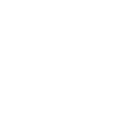
Hardware Platform
Hardware tool is designed for the practical study of neuromorphic technologies and rapid device prototyping. With our platform, users can explore in-memory computing, accelerated matrix-vector multiplication, hardware support for spiking neural networks, and conduct more complex experiments. Our tool enables you to turn ideas into real devices with minimal effort and maximum performance.
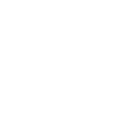
Ecosystem
We are creating not just products but an ecosystem where engineers, researchers, and developers can join forces. Our community is a platform for communication, finding joint projects, learning, and technical support.
Advantages
Light Version
Software
- Masterclasses
- Community
- Simulation Module
- Design Module
- Basic access to libraries
Hardware
- Working with cells
- 8 memristors
Technical Specifications
- ADC - 1 x 8 channels, 12 bit
- DAC - 2 x 2 channels, 12 bit
- Current Measurement - up to 1mA, ±5%
- Resistance Measurement - from 20Ω to 100MΩ, ±5%
- Reading Duration - from 700 μs
- Writing - ±7V, with step of 3mV
- Writing Duration - from 1 μs
Main Version
Software
- Masterclasses
- Community
- Simulation Module
- Design Module
- Testing Module
- Basic access to libraries
Hardware
- Working with cells
- Working with the 32x8 crossbar
- Hardware support for weight matrix transposition
- 32x8 crossbar
Technical Specifications
- ADC - 4 x 2 channels, 12 bit
- DAC - 4 x 8 channels, 12 bit
- Current Measurement - up to 1mA, ±5%
- Resistance Measurement - from 20Ω to 100MΩ, ±5%
- Reading Duration - from 10 μs
- Writing - ±7V, with step of 3mV
- Writing Duration - from 100 ns
Pro Version
Technical Specifications
- Masterclasses
- Community
- Simulation Module
- Design Module
- Testing Module
- Basic access to libraries
- Extended access to libraries
Hardware
- Working with cells
- Working with the 32x8 crossbar
- Working with the 64x64 crossbar
- Hardware support for weight matrix transposition
- 64x64 crossbar
Technical Specifications
- ADC - 16 x 4 channels, 16 bit
- DAC - 8 x 8 channels, 16 bit
- Current Measurement - up to 2mA, ±2.5%
- Resistance Measurement - from 20Ω to 100MΩ, ±5%
- Reading Duration - 10 μs
- Writing - ±10V, with step of 3mV
- Writing Duration - from 50 ns
Cloud Access
Technical Specifications
- Masterclasses
- Community
- Simulation Module
- Design Module
- Testing Module
- Basic access to libraries
- Extended access to libraries
Hardware
- Working with cells
- Working with the 32x8 crossbar
- Working with the 64x64 crossbar
- Hardware support for weight matrix transposition
- On request
Technical Specifications
- ADC - On request
- DAC - On request
- Current Measurement - On request
- Resistance Measurement - On request
- Reading Duration - On request
- Writing - On request
- Writing Duration - On request
Partners
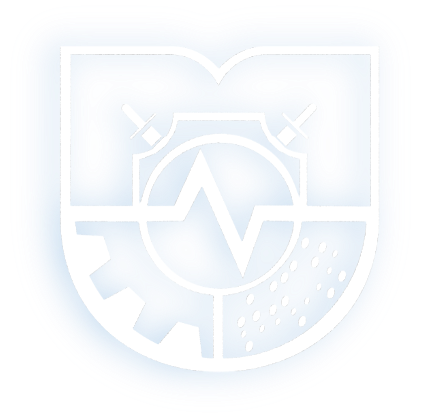
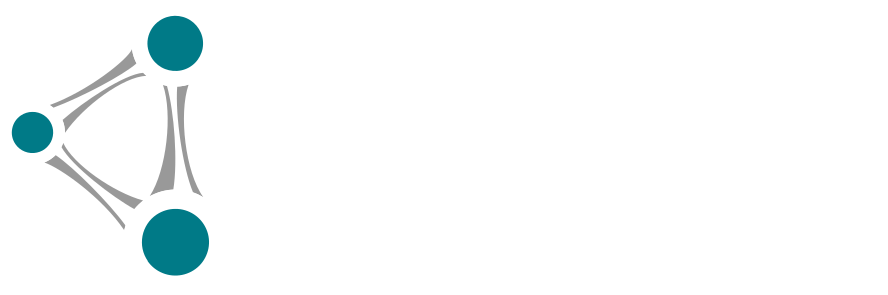
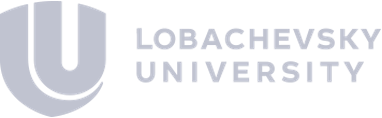

Reviews:
"
The development of MemriLAB makes a decisive contribution to the development of the field of neuroelectronics and neurotechnologies, ensures proactive mastering of new technologies, and promotes scientific and technical results to the market.
"
- A.N. Mikhailov
"
EDA of neuroelectronics is a truly revolutionary product, the need for which is driven by evolutionary reasons! The initiative of Polyketon to solve this problem generates unique domestic solutions for this industry!
"
- S.A. Shchanikov
"
The memristor is currently the most promising electronic component for in-memory computing in the field of neuromorphic devices. The level of technological readiness does not yet allow transitioning to their mass production, so the comprehensive MemriLAB stand for researching memristor matrices is very relevant. Using specialized CAD reduces development time of devices based on them and reduces the level of errors through simulation, testing, and optimization of working parameters.
"
- O.A. Telminov